Definition of Signal and Noise
signal:
Signals are meaningful information with the relevant context in which they’re created or transmitted intentionally to communicate a message, facilitate communication or gain insight. Signals exist across many domains including communication, statistics, and electronics – they’re data or patterns carrying important information which can then be analyzed, interpreted, or decided upon for analysis or decision-making purposes. Signals should not be confused with noise, including unwelcome or irrelevant data that interferes with the reception or interpretation of intended signals.
Noise:
Noise refers to unwanted or irrelevant data which interferes with or disrupts the interpretation, transmission and reception of desirable signals. Noise exists for no discernable purpose or intention and has no intended meaning; its origin lies somewhere outside our grasp and has no set pattern; disturbance, interference and fluctuation from noise can deteriorate signal clarity or quality affecting areas as diverse as communication statistics and biology and degrade signal clarity or quality significantly.
It might come in the form of background noise, static in audio signals, measurement errors in data sets, physiological disorders or environmental influences so its identification and minimization will ensure accurate processing, analysis, transmission and reception for accurate processing analyses and transmission/communication/communiction/exchange of accurate signal processing analyses/communication.
Comparison Table of Signal and Noise
Sure! This table highlights the main differences between noise and signals:
Signal | Noise |
---|---|
Information that is meaningful and has a purpose | Unwanted and irrelevant information |
Intentionally generated or transmit | Nature is random or chaotic |
Contextualizes relevant content | Interferes with or disrupts the interpretation of signals |
Represents valuable patterns or data | Absence of intentionality or purposefulness |
Examples: spoken words, radio waves, trends in data | Example: Background sounds, static audio, measurement error |
Communication and analysis is made easier with this tool | Signal quality and clarity is degraded |
Can be identified and treated for specific purposes | It is important to filter out or minimize the noise for accurate interpretation |
A high signal-to noise ratio (SNR), is desirable | A low signal-to noise ratio (SNR), which indicates poor quality |
Useful for decision-making, research, and problem-solving | Signals for data analysis and communication must be distinguished. |
This is a simplified table. There can be variations in the characteristics and definitions of noise and signals depending on the domain or context where they are used.
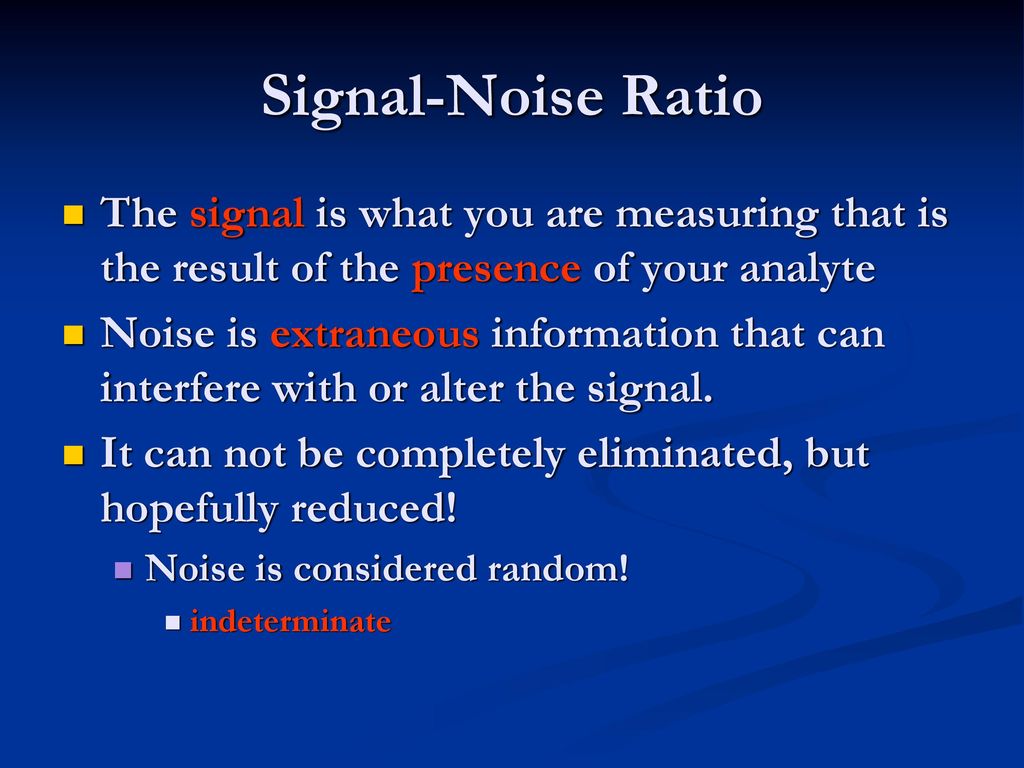
Importance of distinguishing between signal and noise
Recognizing and distinguishing noise and signal is of vital importance across numerous fields and has several important ramifications.
As is so often the case, these efforts to assist our fellow humans have produced only temporary gains – in this instance they merely extended our ability to operate freely as individuals in society as a whole.
Effective Communication: Differencing noise and signal is integral for accurate information transmission through communications systems, making conversation more reliable by decreasing noise emissions and improving signal quality – ultimately guaranteeing that messages reach their destination without misinterpretation or misreception.
Accurate Data Analyses: Statistics and data science depend upon accurate analyses that distinguish meaningful signals from noise in order to interpret and make conclusions with accuracy and reliability. Noise may cause errors or outliers or create misleading patterns which lead to incorrect decisions and false assumptions; when noise is recognized and minimized data analyses become more precise and reliable.
Decision-Making & Problem Solveig: To effectively make decisions and find optimal solutions, filtering out noise can help identify valuable and pertinent information that can guide informed decisions. Those who filter out unnecessary signals to focus on what matters can make informed choices with more confidence while cutting through any noise that arises when making choices can find informed solutions faster.
Scientific Research and Experimentation: To produce reliable and valid research results, it is crucial to distinguish noise from signal. Researchers must carefully plan experiments, control variables, and account for noise when planning experiments to ensure observed effects or signals correspond with whatever phenomenon being investigated.
Signal Processing and Engineering: Signal processing refers to any technique which extracts, filters and enhances signals within noisy data, in order to accurately identify them among noise when creating robust algorithms, efficient communications systems or advanced technology systems.
Sensory perception and human cognition: Our ability to distinguish noise from signal is an integral component of sensory perception in daily life, and is dependent upon brain processes able to prioritize signals over noise – helping us focus on essential information while making sense of our environment.
Reducing noise helps improve communication, data analysis, informed decisions and research findings as well as our overall understanding of our world. Extracting valuable information while decreasing uncertainty allows us to navigate complex systems more successfully.
Understanding Signal
Understanding signals is critical in various fields, from transmission of data and interpretation of interpretation analyses. When trying to comprehend signals, here are a few important considerations you must keep in mind:
As for myself and other members of staff, there has to be something there which allows us to function normally despite any hiccups that might come our way. So far this month hasn’t given anything away!
Definition of Signal: A signal is any meaningful and purposeful piece of data which conveys relevant content for use within specific environments. Signals may be deliberately created or transmitted in order to transmit specific messages, facilitate communications or give insights. Various types of signals may be utilized including acoustic waves, electromagnetic waves and statistical patterns.
Signals have certain distinguishing features that set them apart from noise, including: For instance:
mes mes This signal has been created specifically to convey specific information within a particular setting, with particular functions or meaning being communicated as its central theme.
Relevance: Signals must be relevant to the current problem or situation and offer information helpful in analyzing, interpreting or taking decisions.
Signals can be intentionally created or transmitted from their source or sender. Signals are designed to distinguish themselves from noise and convey messages; as such they’re found across many domains.
Communication signals: In this category are included spoken words, written texts, digital signals and radio waves.
Statistical Signals. Trends, patterns and correlations within data are valuable sources of statistical signals which provide useful data that can be utilized for statistical analysis, modeling and forecasting purposes.
Biological signals: Signals produced by biological systems provide insight into physiological processes. Heartbeats, brainwaves and gene expression levels can all provide clues as to the functioning of body systems.
Process and Analysis: In order to gain an in-depth knowledge of signals, processing and analyzing their contents are often required. Methods like modulation, demodulation and feature extraction may be applied as tools of signal analysis; signal processing uses similar approaches in order to enhance signal quality while extracting meaningful insights.
Signal-to Noise Ratio (SNR): SNR measures the strength and reliability of sound relative to background noise levels.
A higher SNR indicates a strong and reliable signal while lower scores suggest it has become overwhelmed by unwanted background noise, making extracting useful information harder than it otherwise might.
Researchers, engineers and practitioners who possess an in-depth knowledge of signals’ characteristics and appropriate processing methods are adept at understanding, interpreting and exploiting the information contained within them. Signals play an essential role in communications, data processing, scientific research and technological applications alike.
Understanding Noise
Understanding noise is of immense value in numerous fields, as unwanted signals may hinder interpretation, transmission and reception of important data. Here are a few factors you should keep in mind when trying to comprehend noise:
Definition of Noise: Noise refers to data which interferes or disrupts with an intended signal, often at random and chaotic speeds, without any discernible intent or meaning. Noise comes in different forms – random fluctuations, disturbances or interferences can all create unwanted data that interferes with what should be happening and must not.
Noise has several characteristics that distinguish it from signals, including:
Noise can be random or chaotic in its behavior and has no discernable pattern; its unpredictable and chaotic properties often make it hard to distinguish from signals.
Interference: Noise can compromise signal reception, transmission or interpretation and lead to errors, distortions and disruptions which impede efficient communications or analysis. It exists across many fields.
Communication Noise: Noise can come in various forms such as background noise, static audio, electromagnetic interference or distortions to communication channels.
Statistical Noise: Error measurements or random fluctuations that impact statistical analyses or models could all fall under this heading of statistical noise.
Biological Noise: Artifacts or disturbances due to biological measurements, environmental conditions or physiological issues may introduce noise into biomedical applications or research projects, disrupting data or signals and leading to inaccuracy or irrelevancies in results and analysis. Such noise has multiple negative consequences that have an impact on data and signal integrity:
Degraded Quality: Noise can degrade signal quality by adding unwanted distortions or errors, leading to unwanted distortions or errors that muddle the data interpretation or analysis process. Noise may hide patterns within data that should be easily discernable leading to incorrect analysis.
Reduced Reliability: High noise levels can compromise measurement accuracy or reliability, making it harder for researchers to extract useful insights from data sets.
Noise Reduction and Mitigation – Techniques and methods employed to minimize or lessen noise emissions.
Filtering Techniques: Low pass filters may reduce high frequency noise while simultaneously maintaining desired signal outputs. Advanced Algorithms and Signal Processing Techniques can be utilized to detect and reduce noise while maintaining essential signal components. Researchers should utilize appropriate experimental controls in research settings or for data collection purposes in order to reduce noise sources, ensuring more reliable information. This ensures accurate results are collected.
Understanding noise characteristics enables researchers, engineers and practitioners to devise strategies to mitigate noise’s adverse impact, improve signal quality and boost accuracy and reliability in data analysis, communication systems and scientific research projects. By isolating noise from signal, useful information may be extracted in many fields of endeavor.
Conclusion
In many fields and applications, distinguishing noise and signal can be critical. Signals represent information intended to provide clarity or purposeful data exchanged intentionally between parties; noise refers to unwelcome or irrelevant data that interferes with or disrupts interpretation, transmission and reception of signals.
Understanding and recognizing signals allows for improved communication, more precise data analysis and decision making, reliable research results and improved problem solving. Signals offer valuable insight and can provide meaningful data that can then be processed or interpreted further.
Noise degradation of signal quality and its subsequent errors, distortions or misinterpretations is detrimental. A solid understanding of noise characteristics will enable one to effectively identify and mitigate their negative impacts through techniques like filtering or denoising algorithms.
Researchers, engineers, practitioners, and students can improve communication systems by distinguishing noise from signal and making better decisions by analyzing data. Noise management skills develop skills necessary for accurate information transmission, interpretation and problem-solving across diverse domains – this ultimately contributes to effective communications systems, reliable analysis of data sets and scientific progress.